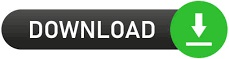
In dryland ecosystems, low vegetation cover is the primary limitation in detecting land surface phenology. Temperate deciduous forests have the most distinct LSP signal, while in other vegetation types LSP is difficult to discern due to combinations of low signal-to-noise ratio between growing and dormant season VI, high snow cover, and low vegetation cover. Yet the use of LSP is limited outside regions with adequate vegetation cover and plants with distinct seasonal change.

Numerous studies measure the short- and long-term LSP trends, linking them to drivers such as weather and climate, land cover change, and disturbances.
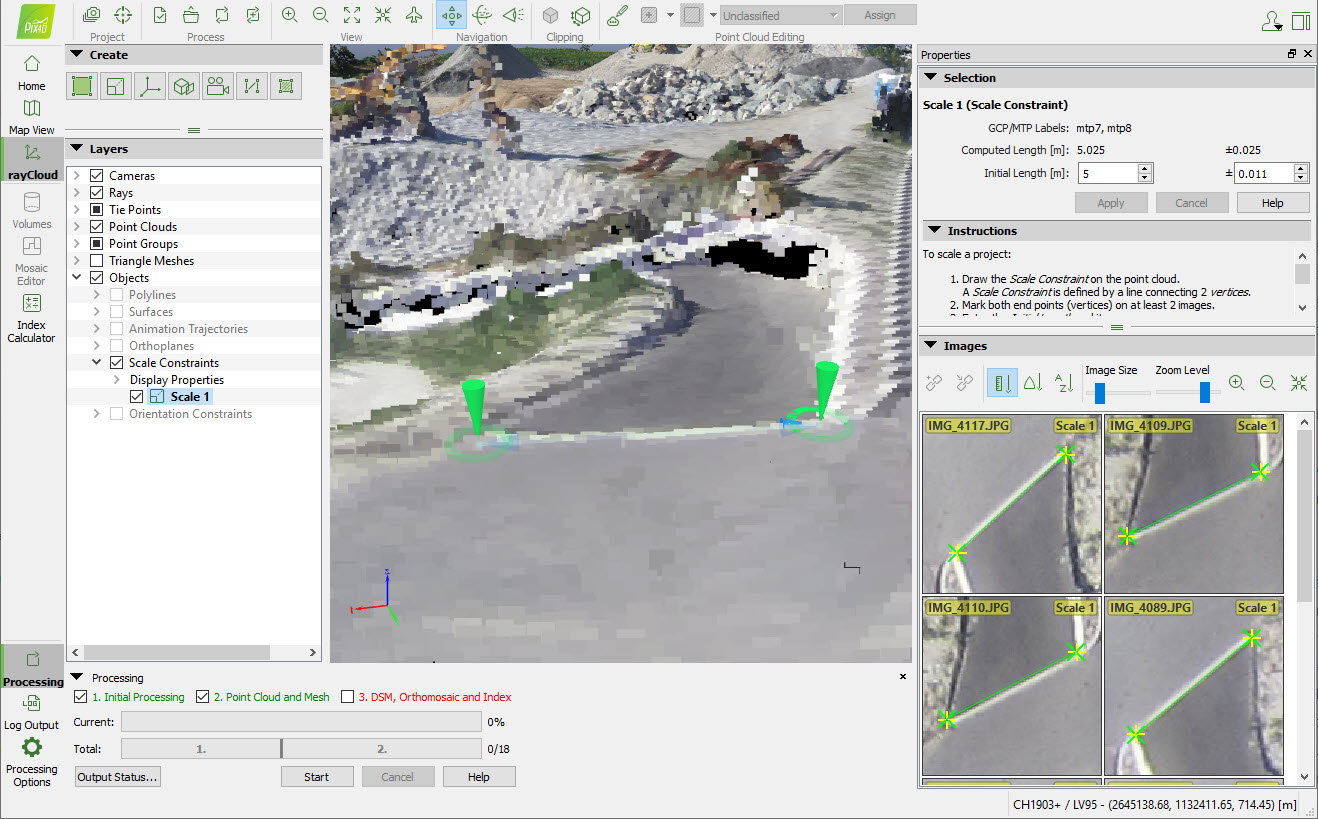
Satellite sensor-derived vegetation indices (VIs) track the progression of green vegetation throughout the year, and from this time series, the seasonal transitions between the dormant and growing seasons are derived. Land surface phenology (LSP) enables ecosystem scale tracking of the drivers and consequences of a changing climate. Drylands thus have the potential to become an exemplary environment for future LSP research. With high-resolution imagery, the open canopies of drylands are beneficial as they allow for straightforward identification of individual plants, enabling the tracking of phenology at the individual level. We showed how high-resolution UAV imagery enables LSP studies in drylands and highlighted important scale effects driven by within-canopy VI variation. Even with adequate cover, biases in phenological metrics can still exceed 20 days and can never be 100% accurate due to VI uncertainty from shadows, sensor view angle, and atmospheric interference. Our evaluation of two algorithms showed that neither performed the best in all cases.

Evergreen plants, with lower seasonal VI amplitude, require considerably higher cover and can have undetectable phenology even with 100% vegetation cover.
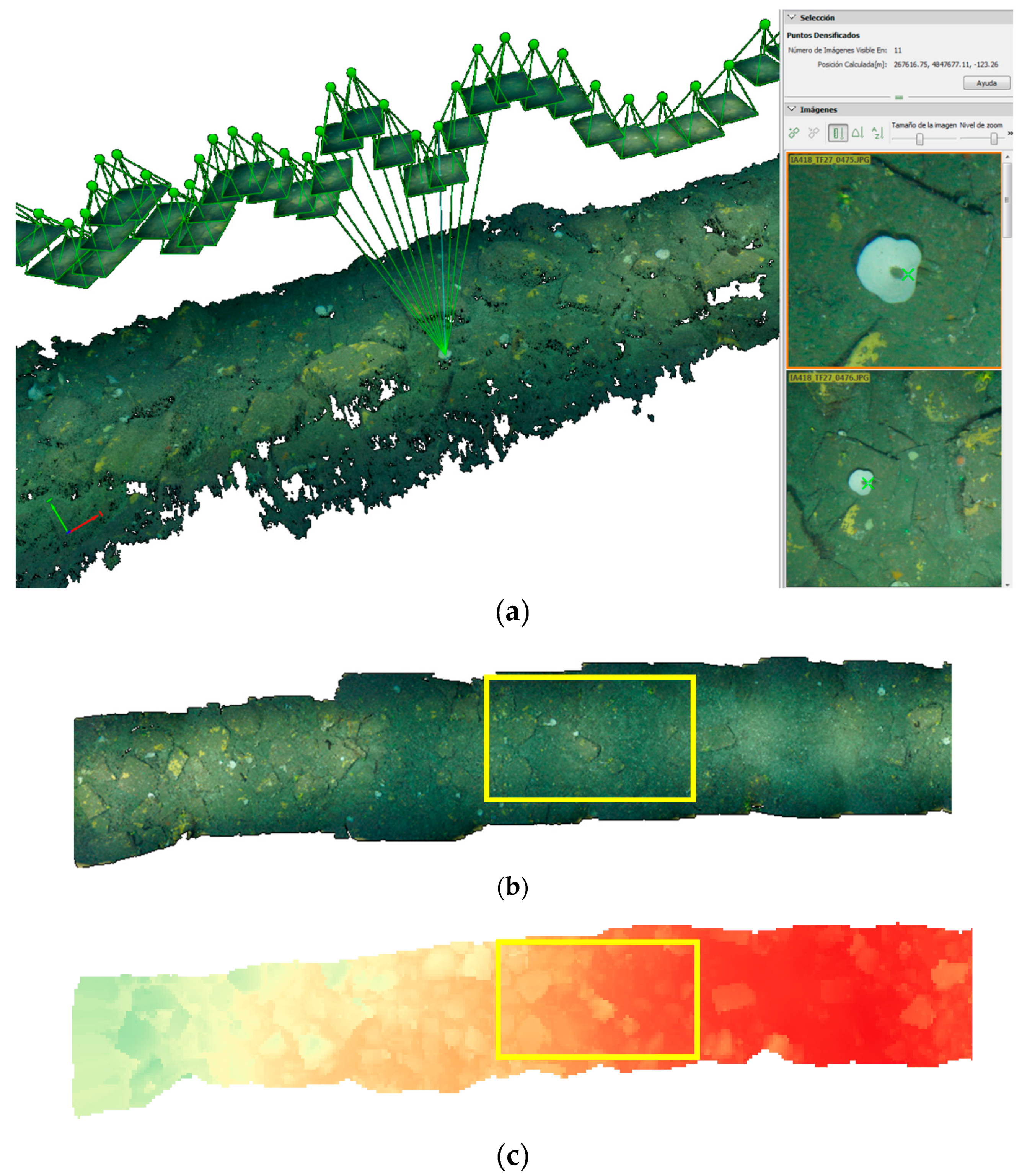
Using simulated data, we found that plants with distinct VI signals, such as deciduous shrubs, can require up to 60% fractional cover to consistently detect LSP. Here, using simulated data and multitemporal unmanned aerial vehicle (UAV) imagery of a desert shrubland, we explore the feasibility of detecting LSP with respect to fractional vegetation cover, plant functional types, VI uncertainty, and two different detection algorithms. Due to the importance of drylands for biodiversity, food security, and the carbon cycle, it is necessary to understand the limitations in measuring dryland dynamics. Land surface phenology (LSP) enables global-scale tracking of ecosystem processes, but its utility is limited in drylands due to low vegetation cover and resulting low annual amplitudes of vegetation indices (VIs).
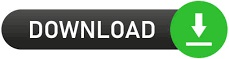